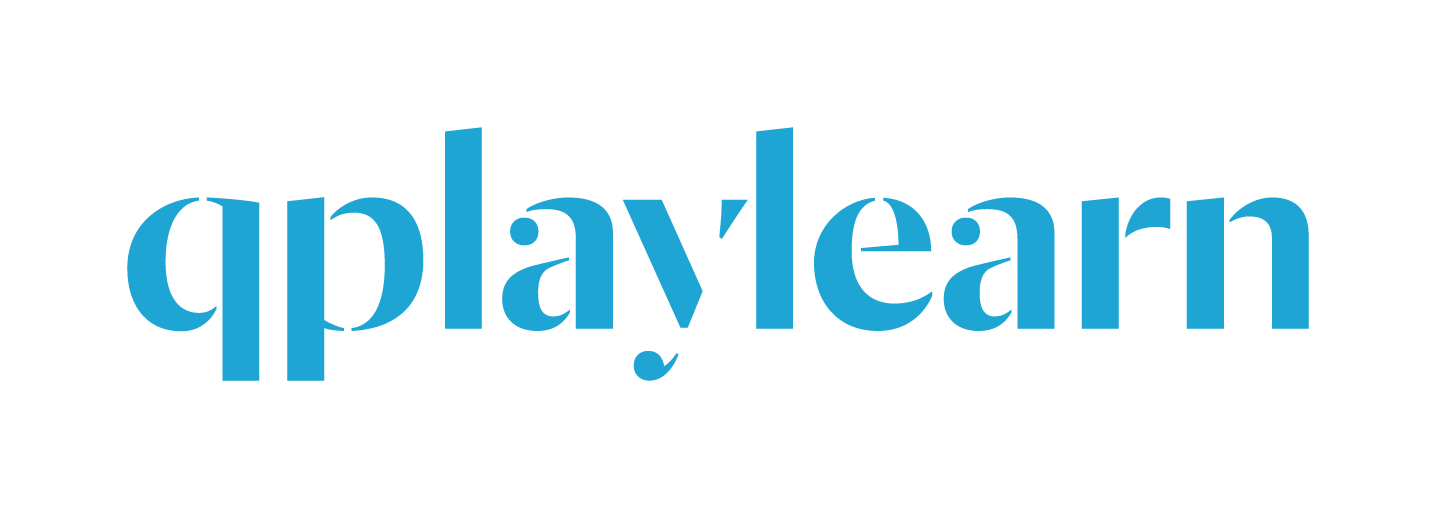
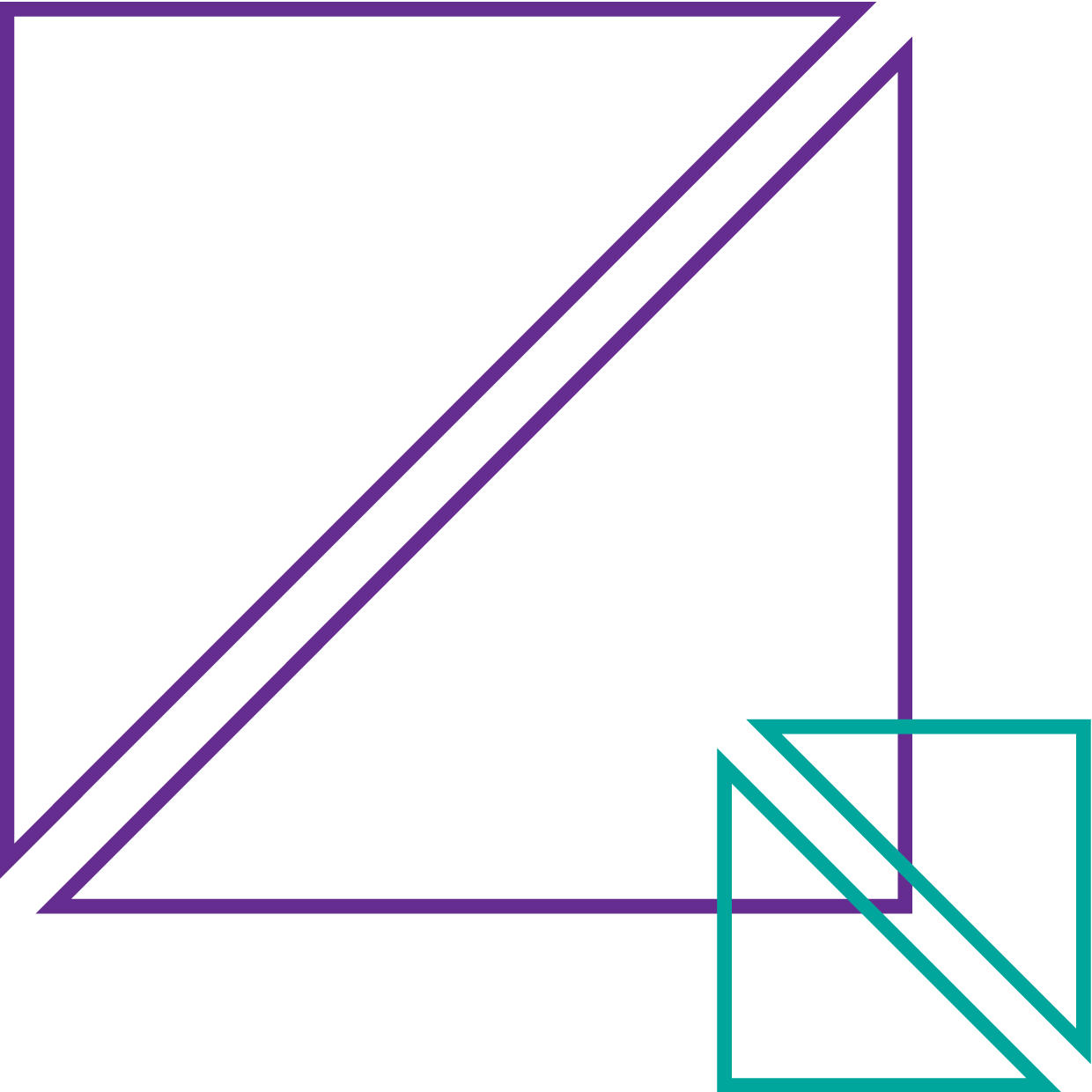
QUEST
Quantum computing for drug discovery
In this entry you will find information on how quantum computing can change the way new drugs are discovered. Start from the section that feels easier or closer to you (play, discover, or learn), and then explore the others to see how your understanding shifts and expands!
“What seems today inconceivable will appear one day, from a higher stand point, quite simple and harmonious.”
—
Max Planck
Play
Challenge for the Algo Team! Can you invent a game inspired by quantum computing in drug discovery?
Breaking Bad blended with an Algo flavour can be considered.
Credits:
Algo Team
Contacts: algothebest@algorithmiq.fi
http://algorithmiq.fi
Discover
Bringing a novel drug from bench to bedside is a complex and challenging task. Pharmaceutical companies and research institutes work hard to create new drugs that are effective and free of unwanted side effects to address the world’s most pressing health problems. In this quest, several factors must be considered, as drugs interact with the cells and the organs of the human body in many ways, and often differently from one patient to another.
An ideal drug disrupts the function of the disease module; it influences the disease mechanism by selectively binding to some (and only some) relevant proteins; it is absorbed, distributed, metabolised and excreted efficiently without toxic effects.
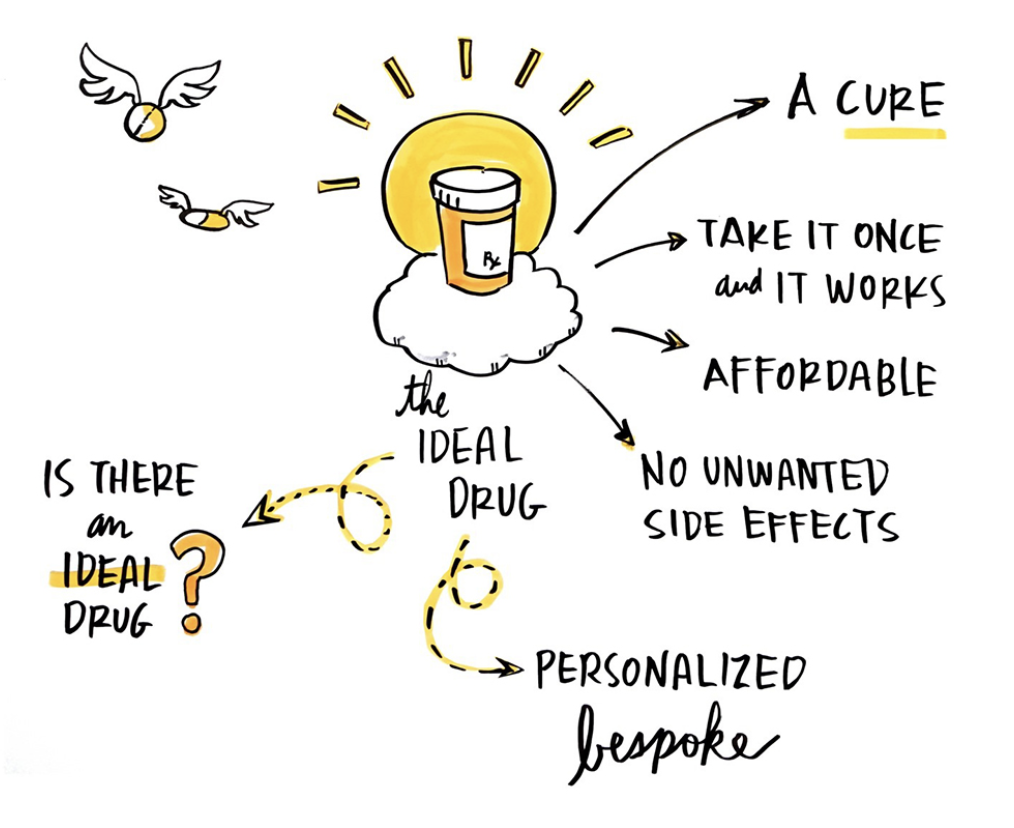
In general, the process of drug development is a long one, very expensive, very complex, and with a very low probability of success. The research phase starts with the identification of a biological target involved in the disease that can be altered by a therapeutic agent. Once the target is identified, a screening campaign commences to identify hit chemical compounds, namely good candidates that could work as therapeutic agents. Then the good candidates need to be optimised in order to understand if they can be finally selected for building the drug and later tested in the clinical trials.
SCHEME - Steps in Drug Development
Moreover, the investment risk in drug development increases over time. In the biopharmaceutical industry, the number of new drugs discovered per billion dollars of R&D funds is decreasing dramatically. Since the 1950s we have gone from about 50 drugs per year to less than 1. It is clear that the pharmaceutical industry is becoming less and less efficient, and that developing drugs is becoming exponentially more difficult.
PLOT
At the beginning this might seem surprising, as the science and technology breakthroughs should allow us to get better and better. But in the biomedical field this is not easy, because in a way the smarter we get, the more risky and complex things become.
Although iterative, often automated, testing methods in the laboratory have produced numerous potential starting points for drug development, there are limitations when it comes to designing and selecting the most promising drug candidates.
So far, screening takes place in the so-called space of known drugs, consisting of tens of millions of already registered and synthesised molecules. But drug hunters could find good candidates among all the potential pharmacologically active molecules, which are estimated to be much more, in the order of 10^63 - to get an idea of how big this number is, watch the video above if you haven't already. Therefore, on the one hand the screening phase is slow because the list is long, but on the other hand we might miss many good candidates hidden in the unexplored regions of the chemical compound space. The final challenge is to have a shortened list with better potential candidates.
To navigate this growing complexity, researchers have to be smart in their choices: in how they design new compounds, build those compounds, and combine screening methods. They need to search where they think they’ll have the most success. And that means the importance of computation has increased.
Acceleration from computing can come on one side through approaches as network medicine (holistic view of the human body as a complex system, crucial for understanding the disease mechanisms and finding better drug targets), on the other side through virtual screening of the known drug-like chemical compounds, with the so called High Throughput drug Screening (HTS).
Discussion on bottlenecks and limitations.
This is where artificial intelligence (AI) and machine learning could come in. Deploying AI to assist chemists in the drug design process holds promise for making better decisions. It is much more efficient than the human mind when it comes to sieving through “big” data, AI generates reproducible results and supports the discovery process by considering many project targets in parallel. But even if promising, AI and machine learning are not the silver bullet.
SCHEME
We have to concede our imperfect understanding of human disease mechanisms. Only when presented with appropriate data will a machine intelligence learn meaningful relationships between drug molecules and their physiological effects. We need more effective models.
Indeed:
- HTS covers a very small region of the CCS (10^7 - 10^8)
- HTS implies series of approximations, low control on the final error
- Tradeoff needed between accuracy and computational efficiency
- Methods limited to non-covalent drug binding
- Drug metabolism too complex for current methods
- AI methods limited from the data quality and representability
Finding drugs with substantial clinical advantage requires efficiently searching in newer and less explored regions of chemical space. Overall, an efficient search in the CCS is not possible with the current methods.
How can QC help and change the way new drugs are discovered? (reorganise in paragraph form)
- Can accurately simulate the electronic structure of molecules
- Can predict chemical properties without training data.
- Can be used to search in CCS.
Conclusion: When will this be possible?
Briefly mention NEAR TERM DEVICES vs FAULT TOLERANT [Challenges in near-term devices & our solutions in the dedicate page]
Techniques on NISQ (VQE) allow to study ground state and excited state properties of the molecules, and a fast optimisation by reiterating the same simulations in different macroscopic conditions in small time scales
Learn
Here you’ll find a summary of the challenges and limitations of the state-of-the-art computational methods used in drug discovery process. Quantum computers could play a disruptive role, enabling efficiently search for novel drugs in new and unexplored regions of chemical compound space.
Contact
Social
Menu
© QPlayLearn 2020-2025